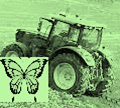
Log in/Register Request a Demo Instruction Manual
A new AI-powered service helping agricultural scientists, farmers, farming companies and experts to make better informed decisions whenever a binary or multi-class prediction or classification is required. For example:
1. Predict wild berry yield levels: Having analysed and measured the soil characteristics of tens of wild berry fields across different regions, and during different past harvest and bloom seasons including texture of soil, structure and depth of soil, organic matter content, density, temperature of soil, level of rainfall, PH, humidity, Nitrogen, Potassium, Phosphorous, Zinc, Iron, Copper, Manganese, slit, sand, Calcium Carbonate (CaCO₃), Cation exchange capacity (CEC), Soil Electrical Conductivity (EC) , Soil organic carbon (OC), Soil organic matter (SOM), the seed density, and the key parameters during the bloom season including total length of frost periods and average temperature of the frost periods after initial bud development, the average wild berry clone size, average number of flowers per plant, the average number of sunny days, density of honey bees in the field, density of bumblebee in the field, density of Andrena bee in the field, density of Osmia bee in the field, the highest record of the upper band daily air temperature, the lowest record of the upper band daily air temperature, the average of the upper band daily air temperature, the highest record of the lower band daily air temperature, the lowest record of the lower band daily air temperature, the average of the lower band daily air temperature, the total number of days, the average of raining days of the entire bloom season and having clarified and recorded the final wild berry yield during those past seasons in those fields at 14 levels (the target and label of prediction) of below 2000 lb per acre, between 2000 lb and 2500 lb, …, between 7500 lb and 8000 lb and above 8000 lb, predict the potential wild berry yield of a new or existing candidate field for the next season (and also present the probability/certainty of the prediction), provided that all the data features (as described above) for that new or existing candidate field are available to Butterfly AI.
2. Predict whether soil is fertile or not: Having measured the levels of pH, Nitrogen, Potassium, Phosphorous, Zinc, Iron, Copper, Manganese, slit, sand, Calcium Carbonate (CaCO₃), cation exchange capacity (CEC), soil electrical conductivity (EC) , soil organic carbon (OC), soil organic matter (SOM) of tens of past soil samples and having correctly verified and labelled those past samples as “fertile” or “not fertile” (the label and target of prediction), predict whether a new soil sample (with all known data features as of above) is fertile or not.
3. Predict and recommend the most suitable crops for different types of soil: Having number of past soil samples and knowing the data features such as texture of soil, structure and depth of soil, organic matter content, density, temperature of soil, level of rainfall, PH, humidity, level of Nitrogen, Phosphorous and Potassium in soil, and knowing from the past successful experiences which crop (rice, maize, chickpea, pomegranate, lentil, apple, watermelon, etc.) was grown most successfully in that type of soil, for a new sample of soil with known data features predict and recommend the most suitable crop to grow,
4. Predict the level of soil moisture during next week: Having number of past soil samples and knowing the data features such as amount of rainfall, the days with sunshine, the month and day of the year, texture of soil, structure and depth of soil, organic matter content, density, temperature of soil, PH, level of Nitrogen, Phosphorous and Potassium in soil, and knowing the level of soil moisture (low, medium or high) from the past successful measurements (the label and target of prediction), for a new sample of soil predict the kevel of soil moisture next week.
Or any other binary or multi-class prediction or classification use cases required by farming and agricultural experts to make decisions.
Log in/Register Request a Demo Instruction Manual